by RadRabbit
Machine Learning Model Evaluation Dashboard
import streamlit as st
import pandas as pd
import numpy as np
st.title('Machine Learning Evaluation Dashboard')
import os
import matplotlib.pyplot as plt
import seaborn as sns
from sklearn.datasets import load_iris
from sklearn.model_selection import train_test_split
from sklearn.ensemble import RandomForestClassifier
from sklearn.metrics import confusion_matrix, classification_report
from PIL import Image
import io
st.title('Built with LazyAI')
st.write("Upload your dataset and run it through pre-trained machine learning models.")
uploaded_file = st.file_uploader("Choose a file")
if uploaded_file is not None:
# To read file as bytes:
bytes_data = uploaded_file.getvalue()
Created: | Last Updated:
Introduction to the Machine Learning Evaluation Dashboard Template
Welcome to the Machine Learning Evaluation Dashboard template provided by Lazy. This template is designed to help you evaluate machine learning models with ease. It features a customizable Streamlit dashboard that allows for interactive elements and real-time visualizations. With this template, you can upload datasets, run them through pre-trained machine learning models, and analyze the results using dynamic charts and confusion matrices.
Getting Started with the Template
To begin using this template, simply click on "Start with this Template" on the Lazy platform. This will pre-populate the code in the Lazy Builder interface, so you won't need to copy, paste, or delete any code.
Test: Deploying the App
Once you have started with the template, you can deploy the app by pressing the "Test" button. This will begin the deployment process and launch the Lazy CLI. The Lazy platform handles all the deployment details, so you don't need to worry about installing libraries or setting up your environment.
Entering Input
After pressing the "Test" button, if the app requires user input, the Lazy App's CLI interface will appear, prompting you to provide the necessary input. Follow the instructions in the CLI to enter the required information.
Using the App
After deployment, you will be able to interact with the Machine Learning Evaluation Dashboard. Here's how to use the interface:
- Upload your dataset using the file uploader.
- Select a pre-trained model from the dropdown menu.
- Adjust the threshold value using the slider.
- Click the 'Run Model' button to process your data and view the results.
The dashboard will display a confusion matrix and a classification report based on the predictions made by the selected model. You can also view dynamic charts that update in real-time as you adjust the parameters.
Integrating the App
If you need to integrate this dashboard into another service or frontend, you may need to use the dedicated server link provided by Lazy. This link can be used to access the dashboard from other tools or services. If the app uses an API, Lazy will also provide a link to interact with the API, and if it's a FastAPI, a docs link will be included for documentation purposes.
Remember, all the necessary steps to run and integrate the template are handled within the Lazy platform. There's no need for additional setup unless specified by the template's requirements.
If you encounter any issues or need further assistance, refer to the documentation provided in the code or reach out to Lazy's customer support for help.
Happy building with Lazy!
Technologies
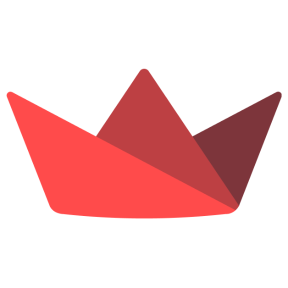
