by Lazy Sloth
FastAPI endpoint for Text Classification using OpenAI GPT 4
import uvicorn
from fastapi import FastAPI
from pydantic import BaseModel, Field
from typing import List
from abilities import llm_prompt
from concurrent.futures import ThreadPoolExecutor, TimeoutError
import json
app = FastAPI()
class Item(BaseModel):
text: str = Field(..., description="The text item to be classified")
class Category(BaseModel):
name: str = Field(..., description="The category name")
def classify_item(item, categories):
try:
prompt = f"Classify the following item: [{item.text}], into one of the following categories: [{', '.join([category.name for category in categories])}]. Respond with only the name of the category, leave empty if nothing matches."
response = llm_prompt(prompt, model="gpt-4", temperature=0.1)
print(response)
try:
response = json.loads(response)
Frequently Asked Questions
What are some potential business applications for this Fast API endpoint for Text Classification?
The Fast API endpoint for Text Classification using GPT-4 has numerous business applications across various industries. Some potential use cases include: - Content categorization for digital media companies - Customer feedback analysis for product development teams - Automated ticket routing for customer support systems - Document classification for legal or financial firms - Market research data categorization for marketing teams
By leveraging the power of GPT-4, businesses can automate the process of categorizing large volumes of text data quickly and accurately.
How can this API improve efficiency in a business setting?
This Fast API endpoint for Text Classification can significantly improve efficiency in several ways: - Automating manual classification tasks, saving time and reducing human error - Enabling real-time classification of incoming data for immediate action - Scaling classification capabilities to handle large volumes of data - Providing consistent categorization across different teams or departments - Allowing for easy integration with existing systems through its API structure
By implementing this solution, businesses can streamline their workflows and make data-driven decisions more quickly and accurately.
What advantages does using GPT-4 for classification offer compared to traditional machine learning methods?
Using GPT-4 for classification in this Fast API endpoint offers several advantages over traditional machine learning methods: - No need for extensive training data or model fine-tuning - Ability to understand context and nuance in text - Flexibility to adapt to new categories without retraining - Capability to handle complex, multi-label classifications - Potential for more accurate classifications due to GPT-4's advanced language understanding
These advantages make the API a powerful tool for businesses that need to classify diverse and evolving text data.
How can I modify the API to include confidence scores for each classification?
To include confidence scores, you can modify the classify_item
and classify_item_multiple
functions to request confidence scores from the LLM. Here's an example of how you might modify the classify_item
function:
python
def classify_item(item, categories):
try:
prompt = f"Classify the following item: [{item.text}], into one of the following categories: [{', '.join([category.name for category in categories])}]. Respond with the category name and a confidence score (0-100) in JSON format, like this: {{\"category\": \"category_name\", \"confidence\": 85}}. Leave empty if nothing matches."
response = llm_prompt(prompt, model="gpt-4", temperature=0.1)
try:
result = json.loads(response)
return {"item": item.text, "category": result["category"], "confidence": result["confidence"]}
except json.JSONDecodeError:
return {"item": item.text, "category": "", "confidence": 0}
except (TimeoutError, Exception):
return {"item": item.text, "category": "", "confidence": 0}
You would need to make similar modifications to the classify_item_multiple
function and update the return structures in the API endpoints.
How can I adjust the concurrency level of the API to optimize performance?
The concurrency level in the Fast API endpoint for Text Classification is controlled by the ThreadPoolExecutor
. You can adjust it by specifying the maximum number of worker threads. Here's an example of how you can modify the API to allow for a configurable number of workers:
```python from fastapi import FastAPI, Query
app = FastAPI()
@app.post("/classify_single") def classify_single(items: List[Item], categories: List[Category], max_workers: int = Query(default=None, description="Maximum number of worker threads")): with ThreadPoolExecutor(max_workers=max_workers) as executor: results = list(executor.map(classify_item, items, [categories]*len(items))) return results ```
In this example, max_workers
is an optional query parameter. If not specified, the ThreadPoolExecutor
will use a default value (typically the number of processors on the machine multiplied by 5). You can experiment with different values to find the optimal concurrency level for your specific use case and hardware.
Created: | Last Updated:
Introduction to the FastAPI Text Classification Template
Welcome to the FastAPI Text Classification Template using GPT-4! This template is designed to help you quickly set up an API that can classify text items into categories using the power of GPT-4. Whether you're building a content categorization tool, a customer support automation system, or any other application that requires text classification, this template will get you started without the hassle of environment setup or deployment concerns.
Clicking Start with this Template
To begin using this template, simply click on the "Start with this Template" button. This will set up the template in your Lazy builder interface, pre-populating the code and allowing you to customize it to your needs.
Initial Setup
There are no environment secrets to set up for this template, as all necessary modules and functionalities are built-in within the Lazy platform. This means you can proceed without any additional configuration.
Test: Pressing the Test Button
Once you have started with the template, you can test the functionality by pressing the "Test" button. This will deploy your application and launch the Lazy CLI. The CLI will prompt you for any required user input, if necessary.
Entering Input
For this template, user input through the CLI is not required as the API endpoints are designed to receive input through HTTP requests. Therefore, you can skip this section and move on to using the app.
Using the App
After testing, Lazy will provide you with a dedicated server link to use the API. Additionally, since this template uses FastAPI, you will also receive a link to the automatically generated documentation for your API endpoints. This documentation will guide you on how to interact with the API, detailing the request formats and available endpoints.
Integrating the App
If you need to integrate this API into an external service or frontend, you can use the server link provided by Lazy. Here's how you can make a sample request to the "/classify_single" endpoint:
`import requests
Replace 'your_server_link' with the actual server link provided by Lazy : url = 'your_server_link/classify_single'
Sample data to classify:
data = {
"items": [{"text": "Sample text to classify"}],
"categories": [{"name": "Category1"}, {"name": "Category2"}]
}
Make a POST request to the API: response = requests.post(url, json=data)
Print the response from the API:
print(response.json())`
And here's an example of what the response might look like:
[
{
"item": "Sample text to classify",
"category": "Category1"
}
]
Remember to replace 'your_server_link' with the actual link provided after deployment. Use the provided server link to integrate the API into your application or service as needed. If your integration requires specific scopes or code placement, ensure you follow the guidelines of the external tool you are integrating with.
By following these steps, you should be able to successfully set up and integrate the FastAPI Text Classification Template into your project. Happy building!
Template Benefits
-
Efficient Content Categorization: This template enables businesses to quickly and accurately categorize large volumes of text data, streamlining content management and organization processes.
-
Scalable Text Analysis: The use of FastAPI and concurrent processing allows for handling multiple classification requests simultaneously, making it suitable for high-volume text analysis tasks in various industries.
-
Flexible Category Management: The ability to define custom categories as API parameters provides businesses with the flexibility to adapt the classification system to their specific needs and industry terminology.
-
Improved Decision Making: By leveraging GPT-4's advanced language understanding, businesses can gain deeper insights into their textual data, supporting more informed decision-making processes.
-
Enhanced Customer Experience: This template can be applied to automate customer inquiry routing, product categorization, or content recommendations, leading to improved customer experiences and satisfaction.
Technologies
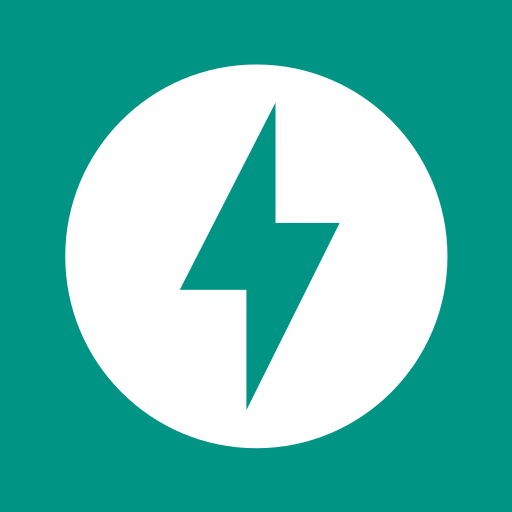