by moonboy
AI UML Generator
import logging
from gunicorn.app.base import BaseApplication
from app_init import create_initialized_flask_app
# Flask app creation should be done by create_initialized_flask_app to avoid circular dependency problems.
app = create_initialized_flask_app()
# Setup logging
logging.basicConfig(level=logging.INFO)
logger = logging.getLogger(__name__)
class StandaloneApplication(BaseApplication):
def __init__(self, app, options=None):
self.application = app
self.options = options or {}
super().__init__()
def load_config(self):
# Apply configuration to Gunicorn
for key, value in self.options.items():
if key in self.cfg.settings and value is not None:
self.cfg.set(key.lower(), value)
def load(self):
AI UML Generator
Created: | Last Updated:
Here's a step-by-step guide for using the AI UML Generator template:
Introduction
The AI UML Generator is a web application that allows users to generate UML (Unified Modeling Language) diagrams from text descriptions using AI. This tool is perfect for software developers, system architects, and anyone who needs to quickly create UML diagrams without manually drawing them.
Getting Started
To begin using the AI UML Generator template, follow these steps:
- Click the "Start with this Template" button in the Lazy Builder interface.
Test the Application
Once you've started with the template, you can test the application:
- Click the "Test" button in the Lazy Builder interface.
- Wait for the deployment process to complete.
- Once deployed, Lazy will provide you with a dedicated server link to access the web application.
Using the AI UML Generator
After the application is deployed, you can start generating UML diagrams:
- Open the provided server link in your web browser.
- You'll see a simple interface with a text area and a "Generate UML" button.
- Enter your UML description in the text area. For example:
Create a class diagram for a library system with Book, Author, and Library classes. Books have titles and ISBNs. Authors have names and can write multiple books. Libraries can contain many books.
- Click the "Generate UML" button.
- The AI will interpret your description and generate a UML diagram.
- The generated UML diagram will be displayed on the page.
Understanding the Generated Diagram
The generated UML diagram will include:
- Classes with their names
- Attributes for each class
- Methods for each class
- Relationships between classes (inheritance, composition, aggregation, or association)
You can use this diagram as a starting point for your software design or documentation.
Integrating the AI UML Generator
The AI UML Generator is a standalone web application, so there's no need for additional integration steps. You can simply use it through the web interface provided by Lazy.
By following these steps, you'll be able to quickly generate UML diagrams from text descriptions using AI, streamlining your software design process.
Technologies
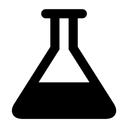
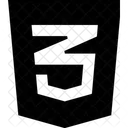

