by Lazy Sloth
FastAPI Backend Server
import logging
from fastapi import FastAPI
from fastapi.responses import RedirectResponse
logging.basicConfig(level=logging.INFO)
logger = logging.getLogger(__name__)
app = FastAPI()
@app.get("/", include_in_schema=False)
def root():
return RedirectResponse(url="/docs")
@app.get("/list")
def list_entrypoint():
some_list = ["data1", "data2"]
return some_list
# Do not remove the main function while updating the app.
if __name__ == "__main__":
Frequently Asked Questions
What types of applications is this Backend Server template best suited for?
The Backend Server template is ideal for building microservices, RESTful APIs, and backend services with minimal frontend requirements. It's particularly well-suited for scenarios where you need to quickly set up a robust API backend, such as for mobile apps, IoT devices, or as part of a distributed system architecture.
How can this Backend Server template improve development efficiency in a business context?
This template significantly enhances development efficiency by providing a pre-configured FastAPI setup. It includes essential components like logging, a basic routing structure, and Pydantic models for data validation. This allows developers to focus on implementing business logic rather than setting up boilerplate code, potentially reducing development time and costs for businesses.
Can the Backend Server template be extended to handle more complex business requirements?
Absolutely. While the Backend Server template provides a streamlined starting point, it's highly extensible. You can easily add more endpoints, integrate with databases, implement authentication and authorization, or connect to external services. The modular structure of FastAPI and the template's organization make it straightforward to scale the application as your business requirements grow.
How can I add a new endpoint to the Backend Server template?
Adding a new endpoint is straightforward. You can create a new function in main.py
or in a separate file and decorate it with FastAPI's route decorators. Here's an example of adding a new GET endpoint:
```python from fastapi import FastAPI
app = FastAPI()
@app.get("/new-endpoint") def new_endpoint(): return {"message": "This is a new endpoint"} ```
If you create the function in a separate file, you can import and include it in main.py
.
How does the Backend Server template handle request validation?
The Backend Server template uses Pydantic for request validation. You can define Pydantic models to specify the expected structure and types of incoming data. Here's an example from the template:
```python from pydantic import BaseModel from fastapi import FastAPI
app = FastAPI()
class Data(BaseModel): field: str
@app.post("/data") def handle_post_endpoint(data: Data): return {"message": f"Received data: {data.field}"} ```
In this example, FastAPI will automatically validate that incoming POST requests to /data
contain a JSON body with a field
property of type string. If the validation fails, it will return an appropriate error response without you having to write explicit validation code.
Created: | Last Updated:
Introduction to the Backend Server Template
Welcome to the Backend Server Template! This template is designed to help you quickly set up a backend service using FastAPI. It's perfect for creating microservices or APIs with minimal frontend requirements. With this template, you'll have a basic server that can handle GET and POST requests, and you'll be able to deploy it effortlessly on the Lazy platform.
Getting Started
To begin using this template, simply click on "Start with this Template" in the Lazy Builder interface. This will pre-populate the code in the Lazy Builder, so you won't need to copy, paste, or delete any code manually.
Test: Deploying the App
Once you've started with the template, the next step is to deploy your app. Press the "Test" button in the Lazy Builder. This will initiate the deployment process and launch the Lazy CLI. The deployment process is handled entirely by Lazy, so you don't need to worry about installing libraries or setting up your environment.
Using the App
After pressing the "Test" button and deploying your app, Lazy will provide you with a dedicated server link. You can use this link to interact with your API. Additionally, since this template uses FastAPI, you will also be provided with a link to the API documentation at "/docs". This documentation will help you understand the available endpoints and how to interact with them.
Here's a sample request and response for the "/list" endpoint:
GET /list
Sample response:
["data1", "data2"]
For the POST request handler, you can use the following sample request:
`POST /your-post-endpoint
Content-Type: application/json
{
"field": "your data"
}`
Sample response:
{
"message": "Received data: your data"
}
Integrating the App
If you need to integrate this backend service with a frontend or another service, you can use the server link provided by Lazy. For example, if you're building a web application, you can make HTTP requests to the endpoints defined in your FastAPI app from your frontend code.
If you need to handle POST requests, you can integrate the provided POST request handler by sending JSON data to the endpoint you define. Make sure to use the correct URL and set the "Content-Type" header to "application/json".
Remember, this template is just the starting point. You can expand upon it by adding more endpoints, request handlers, and integrating it with other services as needed for your specific use case.
That's it! You're now ready to use the Backend Server Template to build and deploy your backend service with ease. Happy coding!
Template Benefits
-
Rapid API Development: This FastAPI-based template allows for quick and efficient development of RESTful APIs, enabling businesses to rapidly prototype and deploy backend services.
-
Scalable Microservices Architecture: The lightweight nature of FastAPI makes this template ideal for building microservices, allowing businesses to create modular, scalable, and easily maintainable backend systems.
-
Automatic Documentation: With FastAPI's built-in Swagger UI (accessible via
/docs
), businesses can provide automatically generated, interactive API documentation, improving developer experience and reducing onboarding time for new team members or API consumers. -
Performance Optimization: Utilizing FastAPI and Uvicorn, this template offers high-performance asynchronous capabilities, allowing businesses to handle a large number of concurrent requests efficiently, which is crucial for high-traffic applications.
-
Easy Integration and Extensibility: The template's modular structure with separate files for different request handlers makes it easy to integrate new features, extend functionality, and maintain clean code organization as the project grows, reducing long-term development costs.
Technologies
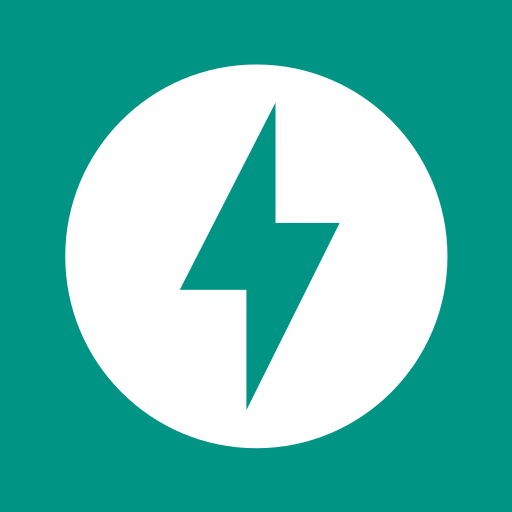
